One property of porous materials is the pattern of their adsorption and desorption curves; in various contexts (e.g. carbon capture), properties of a given material’s soroption curves (e.g. hysteresis) play a major role in the viability of said material for said purpose. While it is possible to generate sorption curves given a material via experiment or simulation, the reverse — generating a material structure that produces a given sorption curve — may be more useful. We accomplish this by first training a CNN to approximate a DFT simulation of the sorption curve, then reversing the architecture and training a generator to output grids representing the structures of porous materials. Since the space of structures has a much higher dimensionality than the space of curves, we augment the input to the generator with a set of latent codes sampled from random noise.
Authors: Yuhai Li, Ziyi Peng, Yuhan Liu, Kevin Li, Mathieu Bauchy
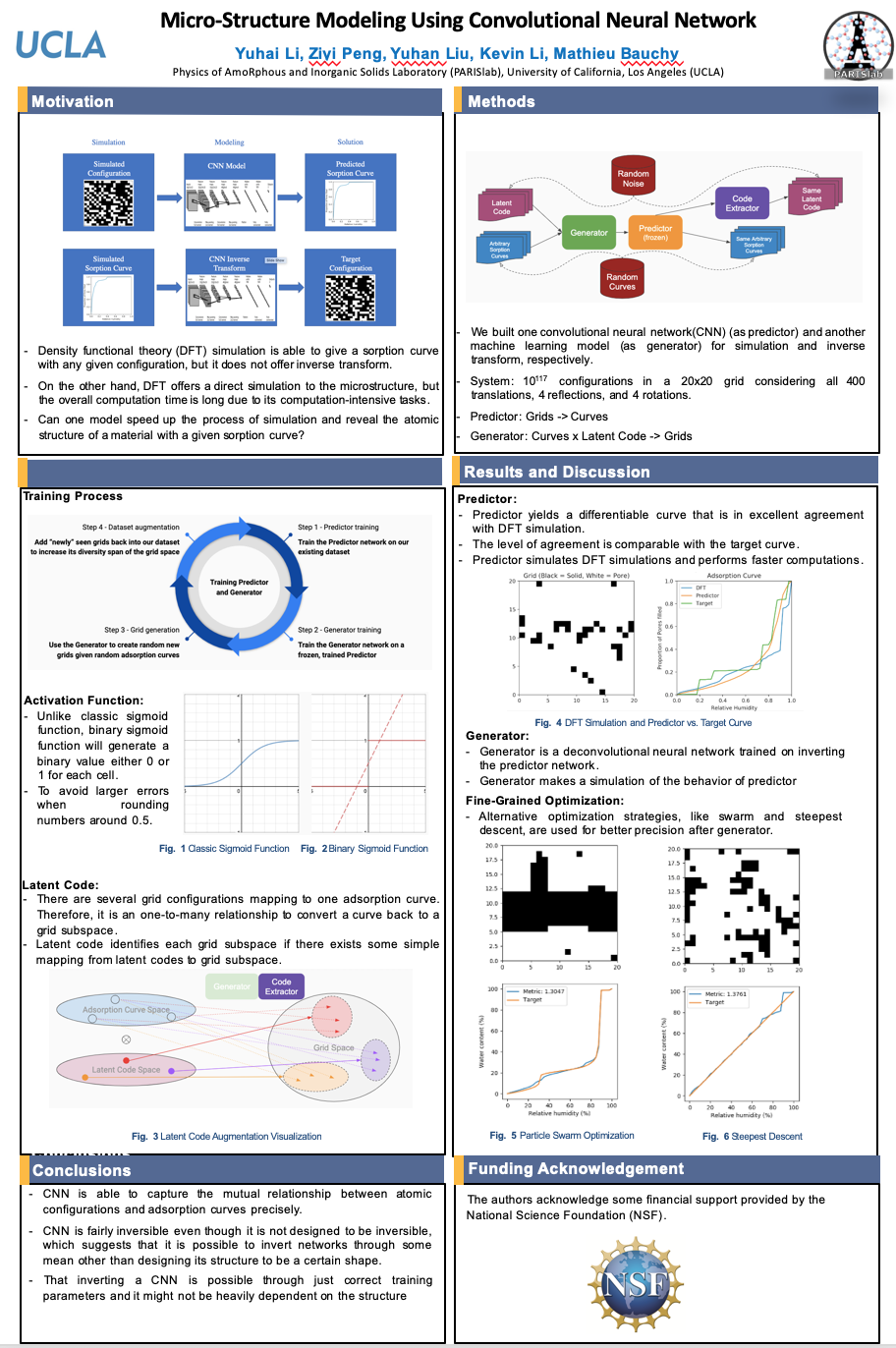